Information usage with a goal.
Information Technology, mathematical statistical foundation.
Decision making, missing foundations.
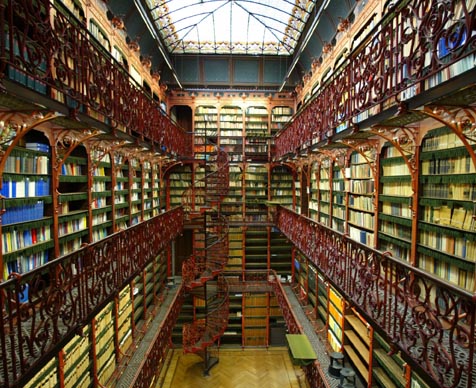
The distance in a flat mapping looks big,
🎭 another dimension:
next door.
 
🔰 the most logical
begin anchor.
This is the part that requires understanding the meaning of information.
The decision makers in an organisation being supported by philosophy theses, using information knowledge and awareness on outcomes impacting others.
 
When looking for the technical communication aspects and A.Turing follow the most logical begin anchor.
Contents
Reference | Topic | Squad |
Intro | Decision making, missing foundations. | 00.01 |
Decide | Goal support decison making. | 10.01 |
FF Statistics | Statistics - Founding Fathers. | 20.01 |
The Lead | Organizing & Leadership. | 30.01 |
The Boss | Human Leadership decisions. | 40.01 |
The Computer | Automated (machine) decisions. | 50.01 |
Greek base | Greek fundamentals decisions. | 01.01 |
Kiss | Divide & Conquer. | 11.01 |
Algebraic | Statistical algorithms. | 21.01 |
Intuitions | Simple decision games. | 31.01 |
Monte Carlo | Uncertainties with predictions & decisions. | 41.01 |
Las Vegas | Uncertainties with machine learning for decisions. | 51.01 |
Progress
- 2020 week:29
- Building up new from scratch again.
Change from the technoloy aspect into philosopy on decision making using and applying statistics technoloy.
- Split in a first conceptual part. Having then number 3,4,5 in a decision process evolvment in changing the approach.
Goal support decison making.
Why Philosophy?
There certainly are many technical aspects of modern information and communications technology (ICT) systems and the associated security architectures.
Indeed, most of the aspect of how to achieve the goals tend to be of a technical nature. However, questions concerning why need not be technical at all. ..
Instead, the goals tend to be more philosophical. They may be framed in a context of moral and ethics, and sometimes in the framework of legislation and societal rules.
{Geir M. Koien 2020 }
 
The need for decision making.
Decison making is necessary when there are relationships with others. As soon a conflict arises the choice is solving that by:
- forcing it in the most powerfull one, survival of the fittest.
There is personal view and others depending on a group interest (chapter squad guild).
- have it solved wihtin in tribe by the leader of the tribe.
Assuming the leader of the tribe is protecting all against other tribes.
I used the words that are common in organsizing agile ICT. A question coudl be: why refering to pre-historic human cultures for implemnting new modern organisations?
🤔 Another question is: why when implementing some process on decison making, then why that is avoided to mention?
 
Ethicals: Volatility uncertainty complexity ambiguity
Vuca world .
The deeper meaning of each element of VUCA serves to enhance the strategic significance of VUCA foresight and insight as well as the behaviour of groups and individuals in organizations. It discusses systemic failuresand behavioural failures, which are characteristic of organisational failure.
- V = Volatility: the nature and dynamics of change, and the nature and speed of change forces and change catalysts.
- U = Uncertainty: the lack of predictability, the prospects for surprise, and the sense of awareness and understanding of issues and events.
- C = Complexity: the multiplex of forces, the confounding of issues, no cause-and-effect chain and confusion that surrounds organization.
- A = Ambiguity: the haziness of reality, the potential for misreads, and the mixed meanings of conditions; cause-and-effect confusion.
Ethicals arround decision making.
Within seeking for new silver bullets organising infromation processing, old question are keeping coming back.
It is generally assumed that collaboration is, in and of itself, a "good thing." "Plays well with others" is high praise from kindergarten onward.
"All of us are smarter than any of us." "The more participation in design, the better." Now, these attractive propositions are far from self-evident.
I will argue that they surely are not universally true. Most great works of the human mind have been made by one mind, or two working closely.
This is true of most of the great engineering feats of the 19th and early 20th centuries. But now, team design has become the modern standard, for good reasons.
The danger is the loss of conceptual integrity in the product, a very grave loss indeed. So the challenge is how to achieve conceptual integrity while doing team design, and at the same time to achieve the very real benefits of collaboration.
[F. Brooks: The Design of Designs, 2010]
Statistics - Founding Fathers.
A huge problem wiht the decison making process is the need for information. What to decide on, which goals, which options to realisations, when to decide, what are the risk, what are the effects, who is involved.
For deleviring information the statistical approach for collecting presenting and predicting is invented and got a defined approach in frameworks.
 
Bayes, 18th century.
Thomas_Bayes (wikipedia) One of the founders for probablity.
Bayesian probability is the name given to several related interpretations of probability as an amount of epistemic confidence " the strength of beliefs, hypotheses etc." rather than a frequency.
This allows the application of probability to all sorts of propositions rather than just ones that come with a reference class. "Bayesian" has been used in this sense since about 1950.
Since its rebirth in the 1950s, advancements in computing technology have allowed scientists from many disciplines to pair traditional Bayesian statistics with random walk techniques.
The use of the Bayes theorem has been extended in science and in other fields.
Laplace, 19th century.
Laplace is more generic. Some of his theory being used at spectral signal processing science. What is called these days "Bayesian" is more likely coming from Laplace.
laplace (wikipedia)
In 1812, Laplace issued his Theorie analytique des probabilitys in which he laid down many fundamental results in statistics.
The first half of this treatise was concerned with probability methods and problems, the second half with statistical methods and applications.
Laplace´s proofs are not always rigorous according to the standards of a later day, and his perspective slides back and forth between the Bayesian and non-Bayesian views with an ease that makes some of his investigations difficult to follow,
but his conclusions remain basically sound even in those few situations where his analysis goes astray.
Fisher, 20th century.
Ronald Fisher (wikipedia)
In 1925 he published Statistical Methods for Research Workers, one of the 20th century´s most influential books on statistical methods.
Fisher´s method is a technique for data fusion or "meta-analysis" (analysis of analyses). This book also popularized the p-value, and plays a central role in his approach.
Fisher proposes the level p=0.05, or a 1 in 20 chance of being exceeded by chance, as a limit for statistical significance, and applies this to a normal distribution (as a two-tailed test), thus yielding the rule of two standard deviations (on a normal distribution) for statistical significance.
The basics on statistics mostly practiced descriptive, the only predcition is extrapolation from a small sample size to a complete population.
Organizing & Leadership.
For a long time the human community is dpendent of leaders and / or advisors on what to do.
A single person is not capable in moving and building hughe enviroments but with many humans in a coordinated approach amazingly in what is possible to achieve.
As soon as the communincation an agreement on the goal is lost all what is doen can collapse faster than it was build up.
 
Real working people feelings.
Decision making a limited philisophy on those aspects.
Decision-making can be regarded as a problem-solving activity yielding a solution deemed to be optimal, or at least satisfactory.
It is therefore a process which can be more or less rational or irrational and can be based on explicit or tacit knowledge and beliefs.
Tacit knowledge is often used to fill the gaps in complex decision making processes.
Usually both of these types of knowledge, tacit and explicit, are used together in the decision-making process.
The decisions are by control, controllers imperators. That is very selected small group of people.
Real working people feelings are like being marionets. Thet are used like machines and commonly excluded from decisions.
Decisions in the ICT devil triangle.
The term elephant test refers to situations in which an idea or thing, "is hard to describe, but instantly recognizable when spotted"
 
🎭 3 Lines from gathering information into decision.
Decisions and ethical challenges.
With decisons and leadship, there are styles of leaderships.
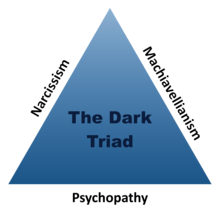
There is optimistic approach to collect as much as possible from positive aspects as the goal. On positive aspects there is openness.
Negative aspects are also existing in the real world. The goal would be to avoid as many of them as possible. On negative aspects there is no openness.
 
Dark triad
All three dark triad traits are conceptually distinct although empirical evidence shows them to be overlapping. They are associated with a callous-manipulative interpersonal style.
- Narcissism is characterized by grandiosity, pride, egotism, and a lack of empathy.
- Machiavellianism is characterized by manipulation and exploitation of others, an absence of morality, unemotional callousness, and a higher level of self interest.
- Psychopathy is characterized by continuous antisocial behavior, impulsivity, selfishness, callous and unemotional traits (CU), and remorselessness.
Dark triad a limited philisophy on those aspects.
A new model of Machiavellianism based in organizational settings consists of three factors:
- maintaining power.
- harsh management tactics.
- manipulative behaviors.
Human Leadership decisions.
The classic leadership is the top down dictation from a human leader. The line of control in humans hierachry and span of control for a pyramid in administration and management.
At the top of the hierarchy the leader everyone in the lines below should obey. The overhead in management in a size equal or more to that of the working ones.
 
T - Tsar replacement Technology.
Tsar Tsar a better desciption for the abbrevation "T" in ICT.
The title tsar is derived from the Latin title for the Roman emperors, caesar.
In comparison to the corresponding Latin word imperator, the Byzantine Greek term basileus was used differently depending on whether it was in a contemporary political context or in a historical or Biblical context.
In the history of the Greek language, basileus had originally meant something like "potentate".
It gradually approached the meaning of "king" in the Hellenistic Period, and it came to designate "emperor" after the inception in the Roman Empire.
As a consequence, Byzantine sources continued to call the Biblical and ancient kings "basileus" even when that word had come to mean "emperor" when referring to contemporary monarchs, while it was never applied to Western European kings, whose title was transliterated from Latin rex, or to other monarchs, for whom designations ("leader", "chieftain") were used.
As the Greek basileus was consistently rendered as "tsar" in Slavonic translations of Greek texts, the dual meaning was transferred into Church Slavonic. Thus, "tsar" was not only used as an equivalent of Latin imperator (in reference to the rulers of the Byzantine Empire, the Holy Roman Empire and to native rulers) but was also used to refer to Biblical rulers and ancient kings.
Decisions on processes.
A simple single process cycle follows the famous PDCA cycle in some way. Just renaming and reordering that, it is like:
- Situation
- Input / Initiatives
- Actions
- Result / Reactions
🎭 4 sides of process phases.
There is a continuous change in view on the objects (vertical/horizontal) and the process actions / transformations (diagonals).
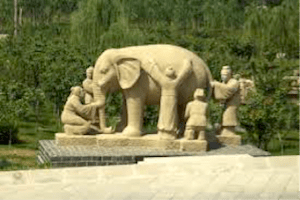
Having a cycle and two different elements, processes and objects, it resembles the electro magnetically theory using the complex imaginary numbers.
Not knowing the Elephant: "is hard to describe, but instantly recognizable when spotted"
Uncertainties and their effects in the results is an ethical aspect. Showing the real situation or something that looks more prettier.
Pareto principle, statistics & decisions.
Go for the low hanging fruit, do not bother you will have up to 20% failures or things going wrong.
🤔 Having 80% going well is good enough ... Is it really good enough?
Pareto-principle
 
Statistical relevant <-> decisions.
🤔 Is the acceptance of 5% (1 out 20 is a mistake / failure) acceptable or not?
😱 Is searching for cases that fulfil the statistical relevance test a correct approach or not?
P-value
In 2016, the American Statistical Association (ASA) published a statement on p-values, saying that
"the widespread use of -statistical significance- (generally interpreted as -p le 0.05-) as a license for making a claim of a scientific finding (or implied truth) leads to considerable distortion of the scientific process"
Automated (machine) decisions.
Whether it is supported in advising human decision makers or full getting fully automated. The dictate of a leader, the Tsar, as the only one responsible for decisions is changing.
New old issues in this change:
- Does the machine replace the human?
- Possible to do things as before?
- Ffully understand the machine and how to use?
It are the common questions when confronted with a disruptive change. The only thing that is sure that is change is inevitable.
 
Control on processes.
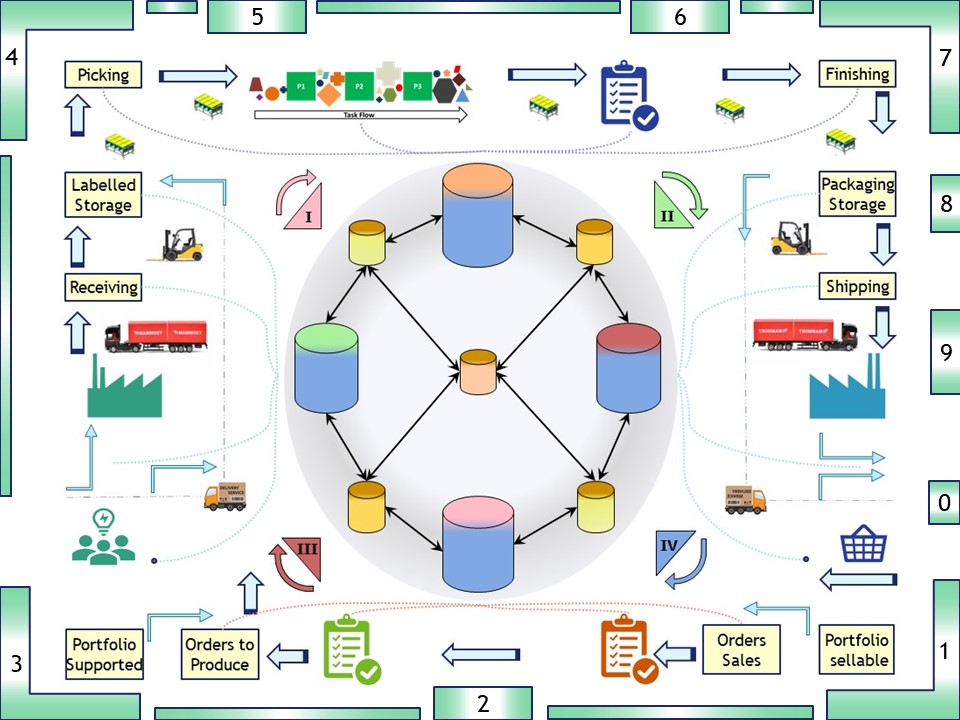
Any of the four process phases might get split up in sub-processes. Each of them running by an own controller, the leader/boss.
 
Before having an abstracted SIAR cycle there is a very detailed version of the business process value stream.
 
In that detailed version the administration and business controls are visible.
The interesting points for control are at the split of handing over responsibilities for a segment of the cycle.
These are the information represented by containers in the diagonals within the control cycle.
Decisions with control on processes.
In a Pull - Push process with the handing over responsibilities the control on the cycle is at those point of change and the overall consolidated one.
Summing up:
- 4 Pull Management
- 3 Demand Control
- 1 Push Management
- 2 Delivery Control
- Consolidated Cycle
🎭 5 Points of process organisation.
The end of classic leadership.
Don Quixote is a leader in a sad setting although his intentions were ethical clear.
Cervantes life an interesting story of conflicts in the world.
When first published, Don Quixote was usually interpreted as a comic novel.
After the French Revolution, it was better known for its central ethic that individuals can be right while society is quite wrong and seen as disenchanting.
In the 19th century, it was seen as a social commentary, but no one could easily tell "whose side Cervantes was on".
Many critics came to view the work as a tragedy in which Don Quixote's idealism and nobility are viewed by the post-chivalric world as insane, and are defeated and rendered useless by common reality.
By the 20th century, the novel had come to occupy a canonical space as one of the foundations of modern literature.
Automated decisions on an ethical base could be a better next evolution for human communities.
Lift, accuracy, confusion matrix
Explainable AI, better understandable ML Machine Learning, automatic decisions is searched for but lacking.
Confusion matrix
In predictive analytics, a table of confusion (sometimes also called a confusion matrix), is a table with two rows and two columns that reports the number of false positives, false negatives, true positives, and true negatives.
This allows more detailed analysis than mere proportion of correct classifications (accuracy).
Accuracy will yield misleading results if the data set is unbalanced; that is, when the numbers of observations in different classes vary greatly.
In this link the important decision to evaluate is whether a pet is a dog or cat. That is of no importance in real life.
In real life the impact on a wrong decision should be another ethic dimension to evaluate.
Missing a single wrong decision that is having a catastrophic impact is better to avoid than many wrong decisions with a little impact.
Understanding decisions with uncertainties & ethics.
Greek fundamentals decisions.
What is known and written handed over in time is for western people most Greek history.
For decisions in ancient times it was common to ask some oracle for the expectations in the future.
 
Pythagoras
The first well known algorithms still referred by this name. It cannot be the oldest way to calculate a surface. Egyptians did that long before these writing and likely others also.
Pythagoras of Samos
Pythagoras ho Samios "Pythagoras the Samian", b. about 570 d. about 495 BC was an Ionian Greek philosopher, mathematician, and founder of the religious movement called Pythagoreanism.
Most of the information about Pythagoras was written down centuries after he lived, so very little reliable information is known about him.
Aristotle & Plato
These old greek philosophers are stating the problem with the analytics. Them meaning and concepts.
theory_of_universals Aristotle to
Platonic realism
Socrates
Plato and Xenophon's dialogues provide the main source of information on Socrates's life and thought.
These writings are the Sokratikoi logoi, or Socratic dialogues, which consist of reports of conversations apparently involving Socrates.
The most famous allegory of the
cave
Any disruptive change altough being the real truth is not automatically an acceptable option for the involved. Decisions on information change by perspectives.
Decisions by leaders in ancient history.
What was challenged in those time did not need any proof. The judgement was done by the majority being empowered.
When the emperor leader as person did not wanted not being personal involved the decision went on other input. The case of Socrates is well described.
 
As easy it was to get condemned just by public opinion sometimes it was evenly easy to get away from anything.
At least the logic and paradox questions started. liar paradox
⚠ The mistake made by Thomas Fowler (and many other people) above is to think that
the negation of "eall Cretans are liars"e is "eall Cretans are honest"e (a paradox) when in fact the negation is
"there exists a Cretan who is honest", or "not all Cretans are liars".
 
💡 The other with this is having an option to ask a question for a smart decision.
Knights and Knaves Improving the uncertainty in an option from 50% into one of 100% certaintity is a remarkable lift.
The important question is: "What question should be asked?".
Divide & Conquer.
Simplify the situation for understanding the options. When understanding the options define soltutions to problems in the situation.
The common approach when not understanding something well, is to get all kind of unrelated things involved. Even worse when it is clear they are unrelated, still keeping those overcomplicating unnecessary artifacts.
 
Keep it stupid simple (KISS)
Simplifying is possible in several ways.
Occam´s_razor
Occam´s razor, Ockham´s razor, Ocham´s razor (Latin: novacula Occami) or law of parsimony (Latin: lex parsimoniae) is the problem-solving principle that "entities should not be multiplied without necessity."
The idea is attributed to English Franciscan friar William of Ockham (c. 1287-1347), a scholastic philosopher and theologian who used a preference for simplicity to defend the idea of divine miracles.
It is variously paraphrased by statements like "the simplest explanation is most likely the right one".
 
KISS principle
The KISS principle states that most systems work best if they are kept simple rather than made complicated; therefore, simplicity should be a key goal in design, and unnecessary complexity should be avoided.
 
⚠ The only question not asked and not answered is what in a situation is simple.
What is a simple step for one perosn is possible felt for another person as very complicated.
Algorithm
Al-Khwarizmi The origin of refering to an algorithmn. An algorithm is an simplified recipe tos solve an known type of problem.
Al-Khwarizmi´s contributions to mathematics, geography, astronomy, and cartography established te basis for innovation in algebra and trigonometry.
His systematic approach to solving linear and quadratic equations led to algebra, a word derived from the title of his book on the subject,
"The Compendious Book on Calculation by Completion and Balancing".
On the Calculation with Hindu Numerals written about 820, was principally responsible for spreading the Hindu-Arabic numeral system throughout the Middle East and Europe.
It was translated into Latin as Algoritmi de numero Indorum. Al-Khwarizmi, rendered as (Latin) Algoritmi, led to the term "algorithm".
Some of his work was based on Persian and Babylonian astronomy, Indian numbers, and Greek mathematics.
 
📚 These not understandable type of alogrithms are basic school maths lessons these days.
How to Solve Quadratic Equations
Just follow the recipe to get the answer.
Statistical algorithms.
There are many statistical concepts described that are usable in some cases. The focus as a pitty is on source-code implementations that are easily copied.
Not need to understand the computional implementations and for too often the limitations where it applicable are ignored.
 
limited list of technical statistics.
The statistical algorithms are in place for a longer period. They should be well known although inventing them by yourself or undertanding their proof of correctness is not that easy.
The usage of all this gets hidden in automated tools doing analyses. The easy mistake is by usingg them incorrectly in worng assumptions.
There are several topics at stats.
⚠ What is done with BI (Business Intelligence) is handing over mainly time series oriented information descriptions to decision makers.
Ignored are all the options in a process were time as an axis component is not that important.
⚠ There are actually dozens of measures of correlation, similarity and dissimilarity. A partial list includes:
- Spearman´s rank correlation
- Kendall´s tau-a and tau-b, Somers´s D
- Chi square test of independence, Gamma
- Phi, Cramer´s V, Contingency coefficient
- Euclidean, Gower, Canberra and Manhattan distance
- Jaccard, simple matching, Tanimoto and Sneath coefficients
- Relative risk and odds ratios
Simple decision games.
The simple games are having counterintuitive evalautions for what is going on. Data driven decisions are suffering form the same kind of assumptions.
 
Choosing and playing random, or not being random.
Three door problem (wiki)
The situation is a brain teaser for the specific conditions into change of chances and proceeding with decisions.
Suppose you're on a game show, and you're given the choice of three doors: Behind one door is a car; behind the others, goats.
You pick a door, say No. 1, and the host, who knows what's behind the doors, opens another door, say No. 3, which has a goat. He then says to you, "eDo you want to pick door No. 2?"e Is it to your advantage to switch your choice?
 
The given probabilities depend on specific assumptions about how the host and contestant choose their doors.
A key insight is that, under these standard conditions, there is more information about doors 2 and 3 than was available at the beginning of the game when door 1 was chosen by the player: the host's deliberate action adds value to the door he did not choose to eliminate, but not to the one chosen by the contestant originally. Another insight is that switching doors is a different action than choosing between the two remaining doors at random, as the first action uses the previous information and the latter does not.
Other possible behaviors than the one described can reveal different additional information, or none at all, and yield different probabilities.
 
The problem is a paradox of the veridical type, because the correct choice (that one should switch doors) is so counterintuitive it can seem absurd, but is nevertheless demonstrably true.
The Monty Hall problem is mathematically closely related to the earlier Three Prisoners problem and to the much older Bertrand´s box paradox.
The "paradox" is in the probability, after choosing a box at random and withdrawing one coin at random, if that happens to be a gold coin, of the next coin drawn from the same box also being a gold coin.
These simple but counterintuitive puzzles are used as a standard example in teaching probability theory. Their solution illustrates some basic principles, including the Kolmogorov axioms.
Choosing and playing random, or not being random.
Rock-paper-scissors
Rock paper scissors is often used as a fair choosing method between two people, similar to coin flipping, drawing straws, or throwing dice in order to settle a dispute or make an unbiased group decision.
Unlike truly random selection methods, however, rock paper scissors can be played with a degree of skill by recognizing and exploiting non-random behavior in opponents.
Uncertaintities with predictions & decisions.
Predicting the future with certaintity is impossible for humans. Simulating and/or doing interpolations are next best options for decisions.
Whether a human does the simulation and/or interpolation or an machine is a matter of game of thrones.
 
Getting in accptable time many choices as options
Monte_Carlo
The underlying concept is to use randomness to solve problems that might be deterministic in principle.
They are often used in physical and mathematical problems and are most useful when it is difficult or impossible to use other approaches.
Monte Carlo methods are mainly used in three problem classes: optimization, numerical integration, and generating draws from a probability distribution.
Random numbers
Generating good random numbers is ever lasting question.
Mersenne_twister
The Mersenne Twister was developed in 1997 by Makoto Matsumoto and Takuji Nishimura. It was designed specifically to rectify most of the flaws found in older PRNGs.
The most commonly used version of the Mersenne Twister algorithm is based on the Mersenne prime 2**19937-1. The standard implementation of that, MT19937, uses a 32-bit word length.
There is another implementation (with five variants) that uses a 64-bit word length, MT19937-64; it generates a different sequence.
A note on why there are shortcomings: (B. A. Wichmann). Another one still present is the seed values being predictable of the system clock.
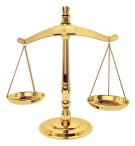
Algorithm AS 183, Hill and Wichmann (1982) and Wichmann and Hill (1982) resulted. It has had a "good innings" but its cycle length of about 7x10**12 must now be considered inadequate.
It has been reported (McCullough and Wilson, 2005) as having failed some tests at a probability level of less than 10**-15, which surely is indicative of a major failing.
Computing developments over the last quarter of a century now make a better version both possible and desirable.
In particular, there does not now seem to be a need for the 16-bit restriction, as 32-bit availability is almost universal.
Benford distribution of numbers
With te conditions of real measures the numbers itself are not random.
Benford´s_law
Benford´s law, also called the Newcomb Benford law, the law of anomalous numbers, or the first-digit law, is an observation about the frequency distribution of leading digits in many real-life sets of numerical data.
The law states that in many naturally occurring collections of numbers, the leading significant digit is likely to be small.
At retail supermakets there is reason why the 9 is in acces for the announced prices.
How a Simple Misconception can Trip up a Fraudster and How a Savvy CFE Can Spot It (acfe)
Dark sides in leader KPI´s
Fame Wealthiness are tempting when in a position to manipulate the prestation figures.
Rogue traders
A rogue trader is an employee authorized to make trades on behalf of their employer (subject to certain conditions) who makes unauthorized trades.
It is most often applied to financial trading, when rogue professional traders make unapproved financial transactions.
In several cases traders have initially made very large profits for their employers, and bonuses for themselves, from trades in breach of the rules, and it has widely been said that employers turned a blind eye to transgressions due to the profits involved.
Enron repeated in another setting, Wirecard scandal.
Uncertaintities with machine learning for decisions.
Machine learning is based on a lot of applied statistics using computational processing capacity just recently became available.
Basic machine learning models are understandable in an understandable process.
Recognizing the subject on images the name and artist of a melody are recently added with "deep learning". The explain ability of those models is less obvious.
 
Getting an accptable option in unknown time
Las_Vegas
Las Vegas algorithms were introduced by Laszlo Babai in 1979, in the context of the graph isomorphism problem, as a dual to Monte Carlo algorithms.
Babai introduced the term "Las Vegas algorithm" alongside an example involving coin flips: the algorithm depends on a series of independent coin flips, and there is a small chance of failure (no result).
However, in contrast to Monte Carlo algorithms, the Las Vegas algorithm can guarantee the correctness of any reported result.
Recognizing cat or dog in a rorsach setting. The result is one the will fulfil algorimtic requiments it is not guaranteeing correct result in the real world perception.
The world of deep learning AI is having results on categorizing images sound and more in a mostly but not absolute correct classifcation.
Usablity classic explainable Machine Learning
The only thing that gets underpinning by using information (data) is the decisions that were done before by "good human feeling".
It is not a negative advice for using machines instead is a positive advice to uses machines wisely.
Qualities:
- clear described goals
- validations options are understandable, possible to process in the same activity.
- dependicies and incomplete / invalid information having correction options.
The problems in doing that were usually showing a bad way of human decision makers. Blaming the machine showing that kind of human issues is human nature.
Usablity Deep Learning
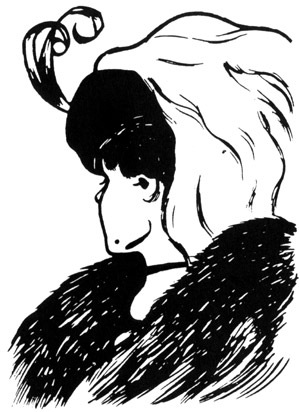
These kind of decisons are partial for another decision. Looking for a vaze of looking persons talking to each other are very different questions.
Asking whether there is a young or old woman and seeing the sae picture having both of them.
The fulfillment by the same image is counterintuitive.
 
What kind of problems are a good condidate for this type ofatomated machine classification decisions?
- clear described goals
- validations options are understandable but NOT possible in the same proces activity.
- dependicies and incomplete / invalid information having correction options.
The validation on being a cat or dog could be eays seen by a human but not by running the same machine model again.
Cleaning up harvested natural goods using machines coud be automated with image recognition. Whether the fall out is well enough segregated is easilty to see by a human but correcing the image selection when not appropiate is a difficult change.
The hyped biometrical recongition in computertechnology I avoided is this list. Probably machines are already better in recognizing humans than humans in the same limitation setting.
© 2012,2020 J.A.Karman