Reference | Topic | Squad |
Intro | Data: describing the process, being processed. | 01.01 |
InfoTypes | Data, Information, Systems everywhere. | 02.01 |
👓 Details InfoTypes | Different types of information (data) | 03.xx |
DocType | Inseperable product documentation layer. | 03.01 |
👓 Lean Administration | Lean administation using ICT. | 02.xx |
Monitor & Archive | Auditing, Logging, monitoring, Retention Policies. | 05.01 |
👓 Informative data | Assembly line,Working cell, machinery & tools. | 04.xx |
BI dwh tech | Preparing data for BI Analtyics. | 04.01 |
👓 BI reporting | Data ware house, Siloed BI. | 05.xx |
What next | Managing data - Transformations. | 06.00 |
| Following steps. | 06.02 |
Progress
THis chapter is fresh. Topics are:
- 2020 week 44
- Coming back to review "data" in reality about information in several layers and different functionality.
- Reordering content in a way there will be room for logging, journalling, auditing, monitoring, data lineage and data retention policies eg functional archiving, disaster recovery.
- 2019 week 44
- Splitting into several pages.
- Adding the lean design and several types of proces improvement. (also been split up.)
- Moving and adjusting the old data page. It will get spread over the sub pages.
Data, Information, Systems everywhere.
Enterprise Engineering, Enterprise Ontology is a good starting point for reviewing what is data about.
Separation of intention and content will create a new field - enterprise engineering - and make that intellectually manageable.
Administrative systems are processing data - information - for al long period using computers.
The options for measuring those processes were limited as computerresources were limited and expensive. That is all changing.
 
Separations of concerns.
Back to: "Enterprise Engineering, Enterprise Ontology" it has a defined pull push circle for processes initiated by the customer.
When adding to a basic process flow the control and actor a circle is appearing.
👓 The figure shows a good seperation of concerns with the focus on the core business.
There a several layers of processing and working on information layers. Those other layers do not have a good description.
The process circle of customer (pull) into procuder (push) in a circle is sensible altought the product flow is rihgt to left where that is normal to have left to right.
How the thinking developed to achieve this is not clear, but in a document dated 2006 that gap of information is closed.
Dividie a bigger issue into smaller ones to have each of them better understood.
Because we struggle with the small sizes of our heads as long as we exist, we need intellectual techniques that help us in mastering the complexity we are faced with (adapted from Edsger W. Dijkstra):
- separation of concerns
- effective use of abstraction
- devising appropriate concepts
Dijkstra successfully separated the concern for correctness from the concern for efficiency in programming and thereby made programming intellectually manageable.
Ways in separation of concerns.
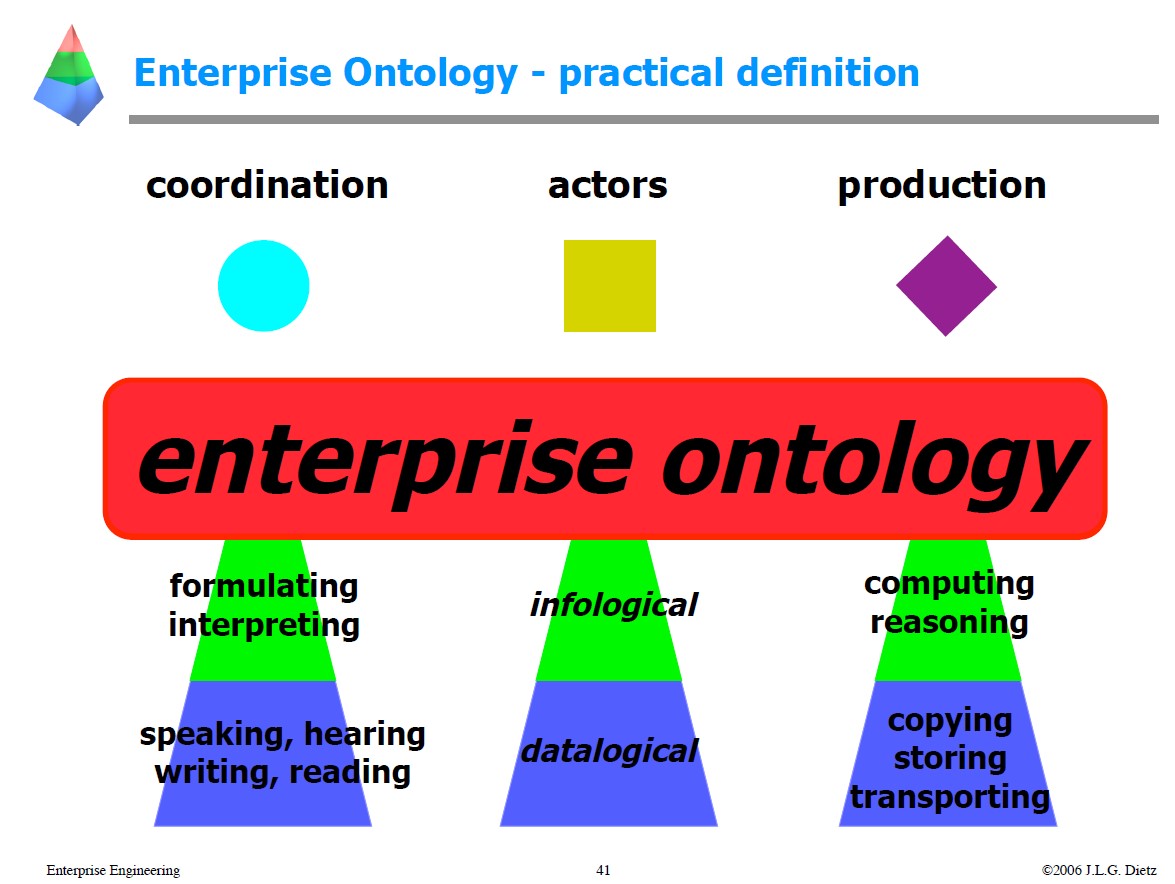
The separtion of concerns is interesting as not having a clear definition on what those are.
Mentioned are: coordination, actor and production.
This Information concern seperation is not according a value stream.
The following is a sensible separtions of concerns when dividing into: shop-floor, administration, enterprise management:
- Coordination (blue cirkel): the business (BPM)
- Actors (brown square): Information Management (IM BIAnl)
- Production (purple diamond): Technology (SDLC)
The red shared horizontal is operational execution. Expectation is everybody is having some shared goal. Reviewing it that way it is a match with the AIM model.
Proces flow value stream.
My personal image for a process flow is full with colors. Blue is for the operational process flow. Green for the assembly manufacturing and yellow for the control (pull). 👓
The process of engineering an enterprise operatinal system.
⚖ 1 Identify customer value.
📚 2 Map the value stream.
⚒ 3 Design logical Flow.
⚙ 4 Establish Pull request. IV - III
⚙ 4 Implement Push delivery I - II
🎭 5 Seek Perfection.
It is: Situation, Input, Activity, Results - Repeat. SIAR an alternative for the well known PDCA cyle (Act = Situation).
Inseperable product documentation layer.
The operational performance of the organisation is having many dependicies. "The core business" is the one that matters. The flow of that activity is a value stream.
Without understanding the structure of information processing no organisation can survive. Enterprise engineerring is trying to understand and optimise that.
 
Demo inverted pyramid.
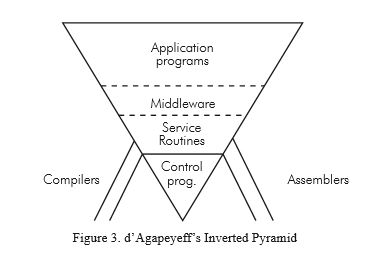
Having this vey old image (ageyff nato69) setting the business on top (most wide used) and technology (small at the bottom) it gives a feeling of a not stable situation.
It is about the separation of concerns: building on technology and adding a "emiddleware layer"e servicing business applications.
 
Reusing this kind of separation and adding the for stages when doing processing gives another idea.
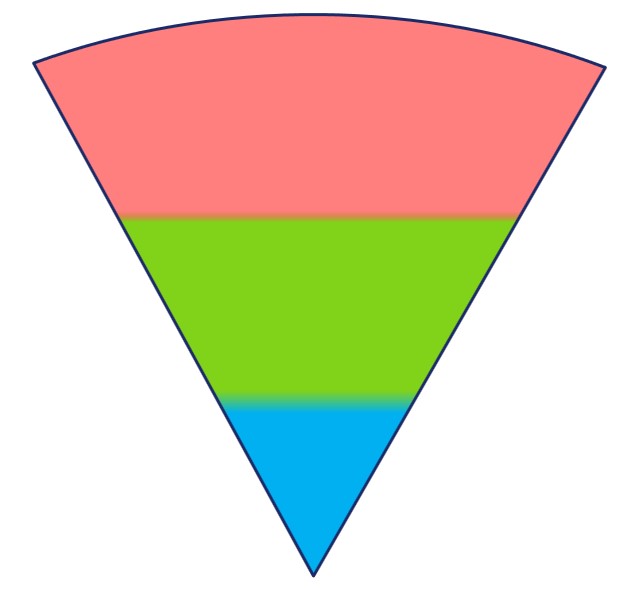
Separtions of concerns: shop-floor, administration, enterprise management:
- Core product (red)
- Administrative information, immediate by time belonging to the core product (green)
eg product properties, list of composing components
- Administrative information, different in time describing the product (green)
eg payment, product warrant, service moments
- Enterprise internal performance information, questions on what did it cost, the profits, customer satisfaction on the product and the service.
Demo into four quadrants cycle.
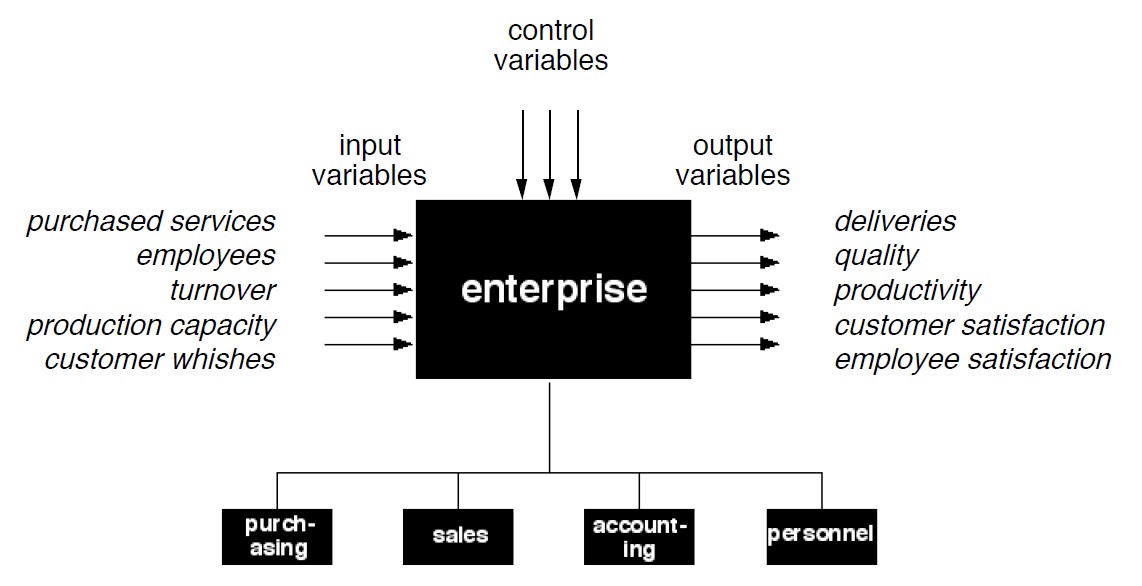
Although Demo is full on the ontology arround the product there is an image showing the flow:
- 🎭 input variables
- ⚙ Transforming by control variables
- 🎯ouput variables
- ⚖ Control: purchasing, sales, accounting etc.
That are for stages of situations these are the edges of four quadrants of a clockwise cycle.
Those four quadrants edges are executed by siloed department lines, seperated by responsabilities.
The interactions with actions between departments are leading to complexity in the overall product flow.
details see link figure 👓
The success factor being mentioned in demo is solving a gap between department lines.
More specific the gap in the ordering (pull backend) and the delivery stage (push frontend) .
Adding Devils triangle & PDCA cycle.
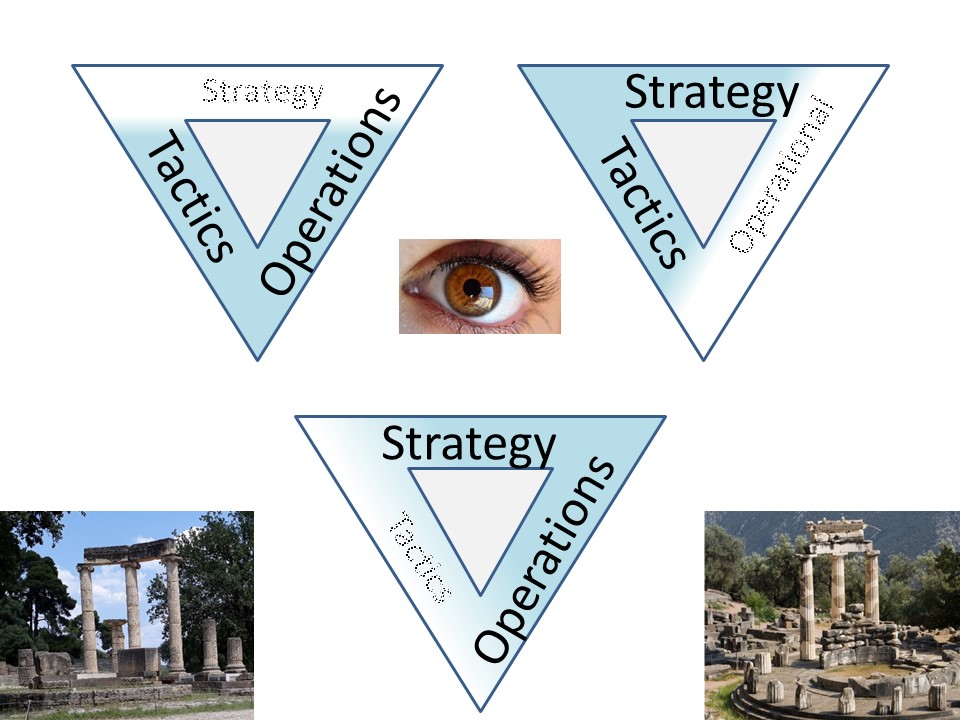
Having those three levels of: Strategy, Tactics, Operations, there is a need to find an optimal balance.
That optimal balance is almost impossible. As soon as two of the three are getting attracted the last one will get out of sight.
 
The one that is most likely to become the underdog an even get lost is the "operations".
That implies the core product, that what is mentioned as mission of an organisation will be lost.
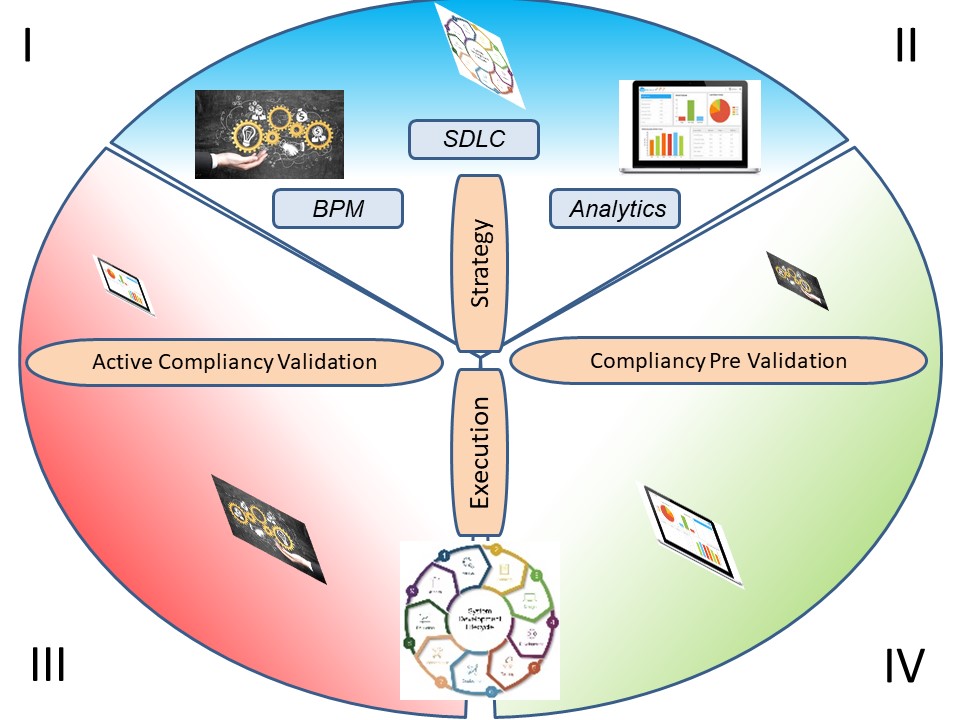
When putting Strategy & Tactics on top Operations at the bottom forming a triangle adding the process life cycle idea to that a process flow in quadrants and segments is building up.
details see link figure 👓
The operations is not at the same level as the two others. Additional representatives are needed at some places to fill the gaps for alignment of all three.
Auditing, Logging, monitoring, Retention Policies.
The most forgotten area of requirements to fulfil for a long term stability, legal auditing, Warranties on the deliverd prodcut, resilience of unexpected events.
The are all not immediate results of an action, the result of the action can be delayed or undetermined by the uncertainty of possibilitie in happening.
 
Archiving.
>
There are many goals fo archiving information (data):
- Warranty for the product after it has been delivered
- Post corrections when the product is not correct
- Legal requirements when there is a case building up or a lawsuit
- Scientific research when the information is relevant enough
details see link figure 👓
💣 The goals are legitim but not being a part of an operational delivery process. The current state of technology could implement it these days by the everlasting growing capacity.
Historically it is missed by the OLTP transactional transformation of administrative processes.
Business Continuity.
The lose of assets can disable an orgnsiation to function. It is risc analysis to what level continuity in what time at what cost is required and what kind of loss is acceptable.
💣 BCM is risc based having visible cost for needed implemtnations but not visible advantages or profits. When being in some fiancial troubles, cost cutting is easily done.
There are many technical options to mitigate an event:
- Having hot stand-by systems. The failover will cover the physical loss of an location but not when there is a logical corruption.
- Cold stand-by systems. The failover will be able to continue for some point in the past. The point is the past being covered as "backup".
There will be information lost by gap in time. Having an active isolated journaling system tath time gap can be minimized at the cost of being longer out of service.
- A dedicated backup-restore approach for any type of an isolated "application"
- An important issue to be aware is waht compnents could get compromised at the same time. Without any isolated verified fall back approach it is possible not being able to recover.
logging monitoring.
Logging is tracing the events in a system, some goals are:
- Knowing who is / has worked on some process. For how long and what resulted as of the actions.
- Regular verifying the state is and what the expectation of those according the administration. Differences to be solved, root cause analyses for avoidance in the future.
This is logical in a physical product approach. 🤔 Why should an administrative product be different? Decoupling will give discrete decision options for operational actions.
- Knowing the required transformations to achieve prescribed results insight can be achieved on the cost/profit.
🚧 using information of what is going on is very useful for optimising (BI Analytics) the processes. For some reason all operational information is copied for that goal.
❗ The Security Operations Centre (SOC) is a spin off with the tasks of evaluating monitoring and reaction on events that possible are compromising integrity of systems and/or breaching information.
Auditing monitoring.
details see link figure 👓
❗ This is better known because external auditors are requiring information for signing annual financial reports.
The limitation is that is easily getting limited to what those auditors are asking. Missing the real reason behind those questions, the goal being just making that singing off happening.
Information (data) relationsships.
Is used at:
👓
threats for data & tools Proces Life Cycle.
👓
Release management SDLC - release management.
👓
Business Intelligence,Analytics .
Details to be found at:
👓
Meta
👓
Math Software engineering.
The Figure with quadrants in a shop-floor operational process is not one found in any design.
It is one that is a logical operational result by separations responsibilities. Going for a data lineage, product pull tracing, in the process circle this is unavoidable.
It is described at the
devops data. Easy switching in he top.
Preparing data for BI Analtyics.
This area is onerwhelmed by a lot of attention in using tools doing some standard approach of gathering information.
At the same time all that what is claimed and said is missing the alignment to the goals of the orgnsiations all too often.
 
Data from operational systems.
Preparing data for analytics has started in the 80´s.
Copying that to another dedicated location (ODS).
Reading transforming log and reports into useful information.
 
Still as a dedicated solution for analytics. The DWH being build with a new data model "Data Vault".<
The total execution time and lack of test approaches like regression tests result into a costly difficult to manage environments.
 
Steps in the proces:
- Retrieving Operational, (copy data) dropping it: ODS Staging.
- Loading into a new database (copy data), into the edw.
- Extracting (copy again), what is necessary loading into data-marts, cubes.
- From those marts cubes creating (copy data) the management information.
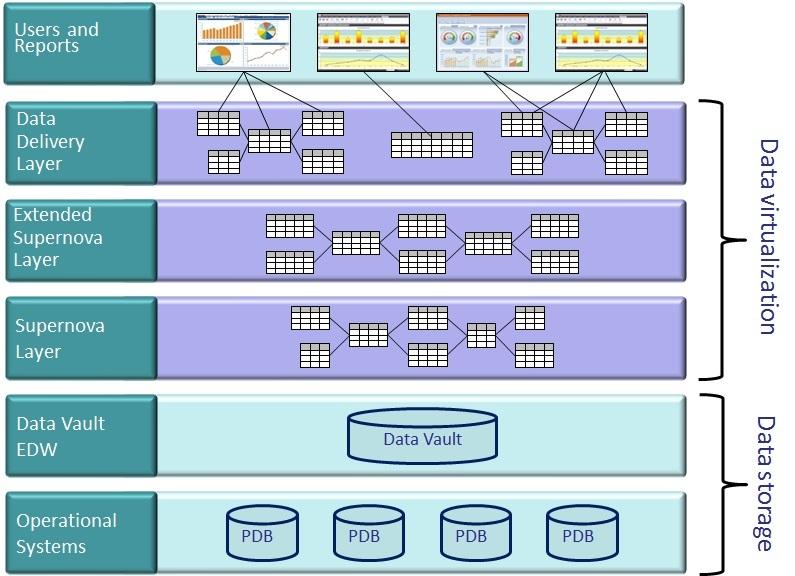
BI datavirtualization.
 
Almost all data in BI is about periods. Adjusting data matching the differences in periods is possible in a standard way.
 
The data virtualization is on the data vault DWH dedicated for BI reporting usage. It is not virtualization on the ODS, or original data sources.
Combining the data transfer, microservices, archive requirement, security requirements and doing it like the maturity of physical logistics goes into the direction of a centralized managed approach.
 
💡 Reuse of standard solutions in a standard way has always been promoted as better and cheaper. Why would we not do that for data ware housing, data transport? EDWH-3.0.
A vmap approach, coordinated planned actions of different topic lines to build a new manufacturing place environment.
details see link figure 👓
💡 Reinvention of patterns. The improvement of a flow shop goes by little innovative steps. A new one with all being rebuild isn't a flow at all.
When a Job shop and project shop are better in place for a situation, why not copy those to ICT?
Managing data - Transformations
💣 Analysing data is requiring real operational production data. Building decisons on faked information would generate very wrong results.
Whether it is basic analytics doing reporting or automatized ML it is =level 3= (orange).
 
Avoiding waste - Lean
Producing more than what is needed is a waste. Lean production is lean especially because it produces only what is needed.
- Any type of transportation is waste. The less you transport, the better.
- Avoid unnecessary movements. Arrange everything so the worker has to move as little as necessary to assemble the product.
- Waiting: This refers primarily to people waiting. Not quite as important but also included are machines waiting.
- Over-processing refers to inclusion of additional features, parts, processes, or other things that the customer does not need and hence is not willing to pay for.
- Defects and Rework: Any product that does not satisfy the requirements and has to be reworked or thrown out.
- Inventory: Any kind of material you have but do not work on right now is a waste.
- Producing more than what is needed is a waste. Lean production is lean especially because it produces only what is needed..
For some reason all of these waste types can be seen as common daily practice using ICT and administrative systems.
Although claims are made they would doing something lean, agile at ICT there is a different perception by the involved.
Enterprise Ontology
The context of "Enterprise Ontology" is far beyond that of just "data - information" it is about most aspects of an enterprise, organisation. Not just the data - information.
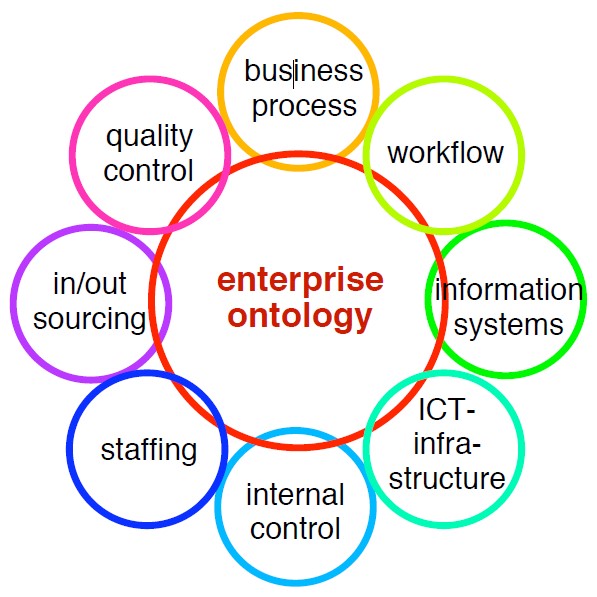
Conclusions in that 2006 refrence are:
- Enterprise Ontology reveals the essence of an enterprise in a comprehensive, coherent, consistent, and concise way, while reducing complexity by well over 90%.
- Enterprise Architecture guides the (re)design and (re)engineering of an enterprise such that its operation is compliant with its mission and strategy, and with all other laws and regulations.
- The new point of view of EE is that enterprises are purposefully designed and engineered social systems.
The reference to a social system states that most issues are based "organisational" not by technical matters.
Following steps
These are design data concepts, others:
Meta, describing data 👓 next
bianl previous, business Intelligence & Analytics.
Others are operational realisations: 👓
data meta math
© 2012,2020 J.A.Karman