Design Data - Information flow
information, data: enterprise core objects.
Data, gathering information on processes.
The data explosion. The change is the ammount we are collecting measuring processes as new information (edge).
📚 Information questions.
⚙ measurements data figures.
🎭 What to do with new data?
⚖ legally & ethical acceptable?
🔰 Most logical
back reference.
Contents
Reference | Topic | Squad |
Intro | Data, gathering information on processes. | 01.01 |
data meaning | Enterprise engineering, valuable processing flows. | 02.01 |
structuring | Information - data - avoiding process fluctuations. | 03.01 |
inbound dwh | Edwh 3.0 - Data: collect - store - deliver. | 04.01 |
outbound dwh | Patterns by changing context, changing technology.
| 05.01 |
What next | Change data - Transformations. | 06.00 |
| Combined pages as single topic. | 06.02 |
Combined pages as single topic.
🕶 info types different types of information
🚧 info types different types of data
👓 Value Stream of the data as product
👓 transform information data inventory
👓 data silo - BI analytics, reporting
Progress
- 2020 week:44
- Redesigned the content while using serveral generic concepts Lean and demo after having the bianl having redone.
- Removed duplicates in old content.
- 2019 week:47
- Redesigned as result of the needed split.
- Focus on the ideas EDWH 3.0 as enterprise service.
d´Agapeyeff&Acute Inverted Pyramid.
Enterprise engineering, valuable processing flows.
Everybody is using a different contact to the word "data". That is confusing when trying to do something with data. A mind switch is seeing it as information processing in enterprises.
As the datacentre is not a core business activity for most organisations there is move in outsourcing (cloud SAAS).
 
Information - data - avoiding process fluctuations.
Engineering a process flow, then at a lot of point there will be waits.
At the starting and ending point it goes from internal to external where far longer waits to get artefacts or product deliveries will happen.
Avoiding fluctuations having a predictable balanced workload is the practical solution to become effciënt.
💣 The role of the EDW 3.0 is enterprise operationals value stream. It is not something being reserved for reporting purposes (BI AI).
THe EDWH 3.0 Logistics as basic central pattern.
Having a inbound area the validation of goods, infomation, is done.
At the manufacturing side are the internal organisation consumers. Not only for a dashboard to be used by managers but all kind of consumers including operational lines.
The two vertical lines are managing whos has acces to what kind of data, autorized by dataowner, registered data consumers, monitored and controlled.
The confidentiality and integrity steps are not bypassed with JIT (lambda).
- ⚖ What is coming in, is expected and valid by administration purchases.
- ⚖ What is coming in, has an internal responsible party with a budget for storage.
- ⚖ What is going out, is deliverd to autorised consumers.
- ⚖ What is going out, has an internal responsible party with a budget for delivery.
Edwh 3.0 - Data: collect - store - deliver.
Processing objects, collecting information and delivering goes along with responsibilities.
It is not sexy, infact rather boring. Without good implementation all other activities are easily getting worthless. The biggest successed like Amazon are probably more based in doing this very well than something else.
The Inner Workings of Amazon Fulfillment Centers
 
Focus on the collect - receive side.
There are many different options how to receive information, data processing. Multiple sources of data - Multiple types of information.
- ⚒ Some parties are reliable predictable available.
With internal systems this is usual.
- Internal Sub products
- Administration (not possible as physical)
- Operational factory chain
- ⚒ Other parties are less reliable, predictable having less availability.
With external systems this is usual.
- No dependency
- Internal dependency, prescriptions outsourced subtask
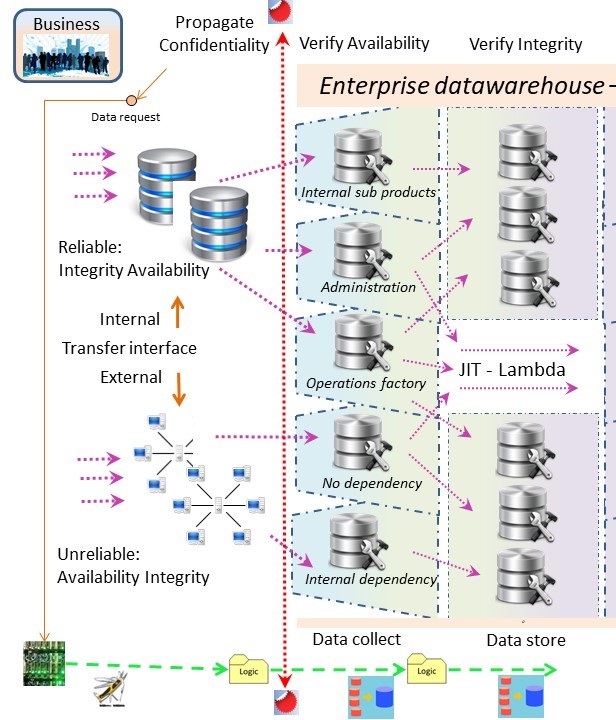
In a picture:
 
A data warehouse should be the decoupling point of incoming and outgoing information.
 
A data warehouse should validate verify the delivery on what is promised to be there.
Just the promise according to the registration by administration, not the quality of the content (different responsibility).
Focus on the ready - deliver side.
A classification by consumption type:
- ⚒ Operations For goals where standard systems are not appropriate or acting as an interface for not coupled systems. 💰 Results are input for other data consumers. Sensitive data allowed (PIA).
- ⚒ Archive of data - information not anymore available in operations, only for limited goals and associated with a retention period. ⚖
- ⚒ Business Intelligence (reporting). Developing and generating reports for decision makers. Possible as usage of analytical tools with DNF. ✅ Sensitive data is eliminated as much is possible.
- ⚒ Analytics Developing Machine Learning. ❗ This is: ALC type3. Sensitive data is eliminated as much is possible.
- ⚒ Analytics, Operations Machine Learning. ❗ This is: ALC type3. Sensitive data may be used controlled (PIA). Results are input for other data consumers.
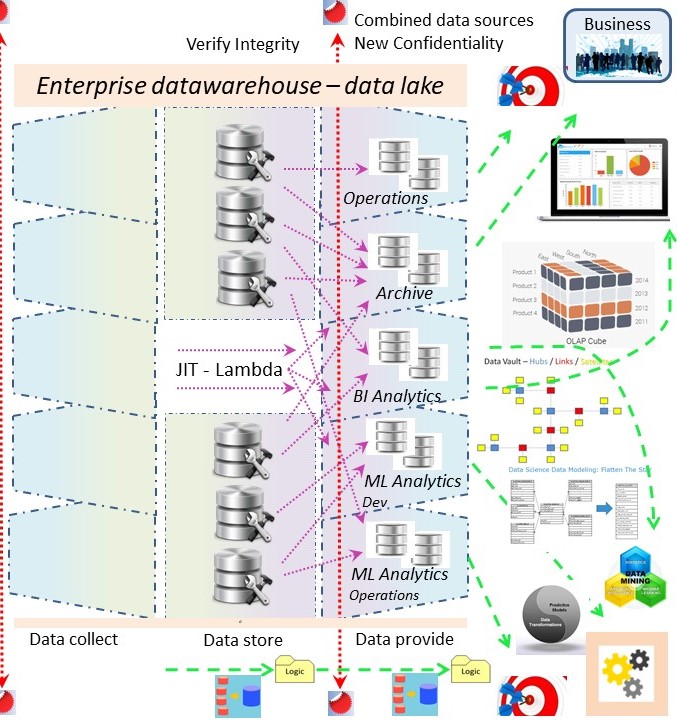
In a picture:
 
There are possible many data consumers.
It is all about "operational" production data" - production information.
 
Some business applications only are possible using the production information.
Patterns by changing context, changing technology.
Common used ICT patterns processing information.
For a long time the only delivery of an information process was a hard copy paper result.
Deliveries of results has changed to many options. The storing of information has changed also.
 
The technical solutions as first process option.
Sometimes a simple paper note will do, sometimes an advanced new machine is needed.
It depends on the situation. A simple solution avoiding the waste is lean - agile
Optimization Transactional Data.
An warehouse does not content structuring it must be able to locate the wanted content structured. Delivering the labelled containers efficient >
Optimization Transactional Data.
The way of processing information was in the old day using flat files in the physical way. Still very structured stored and labelled.
In the modern approach these techniques still are applicable although automated hidden in a RDBMS .
Analytics & reporting.
The "NO SQL" hype is a revival of choosing more applicable techniques.
It is avoiding the transactional RDBMS approach as the single possible technical solution.
Information process oriënted, Process flow.
The information process in an internal flow has many interactions input, transformations and output in flows.
⚠ There is no relationship to machines and networking. The problem to solve those interactions will popup at some point.
⚠ Issues by conversions in datatypes, validations in integrity when using segregated sources (machines) will popup at some point.
The service bus (SOA).
ESB enterprise service bus
The technical connection for business applications is preferable done by a an enterprise service bus.
The goal is normalized systems.
Changing replacing one system should not have any impact on others.
Microservices with api´s
Microservices (Chris Richardson):
Microservices - also known as the microservice architecture - is an architectural style that structures an application as a collection of services that are:
- Highly maintainable and testable.
- Loosely coupled.
- Independently deployable/
- Organized around business capabilities.
The microservice architecture enables the continuous delivery/deployment of large, complex applications. It also enables an organization to evolve its technology stack.
Data in containers.
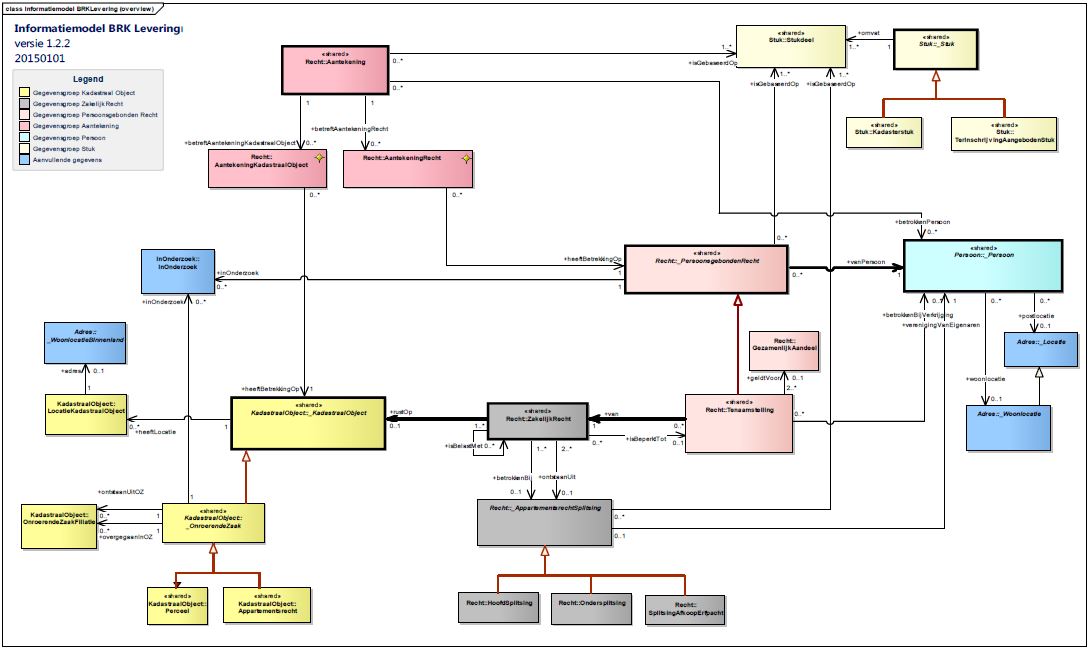
Data modelling using the relational or network concepts is based on basic elements (artefacts).
An information model can use more complex objects as artefacts. In the figure every object type has got different colours.
The information block is a single message describing complete states before and after a mutation of an object. The Life Cycle of a data object as new metainformation.
Any artefact in the message following that metadata information.
⚠ This is making a way to process a chained block of information. It is not following the blockchain axioma´s.
The real advantage of a chain of related information is detecting inter-relationships with the possible not logical or unintended effects.
Optimization OLTP processes.
The relational SQL DBMS replaced codasyl network databases (see math).
The goal is simplification of online transaction processing (oltp) data by deduplication and
normalization (techtarget)
using DBMS systems supporting ACID
ACID properties of transactions (IBM).
These approaches are necessary doing database updates with transactional systems. Using this type of DBMS for analytics (read-only) was not the intention.
normalization (techtarget, Margaret Rouse )
Database normalization is the process of organizing data into tables in such a way that the results of using the database are always unambiguous and as intended.
Such normalization is intrinsic to relational database theory.
It may have the effect of duplicating data within the database and often results in the creation of additional tables.
ACID properties of transactions (IBM)
- Atomicity
All changes to data are performed as if they are a single operation. That is, all the changes are performed, or none of them are.
For example, in an application that transfers funds from one account to another, the atomicity property ensures that, if a debit is made successfully from one account, the corresponding credit is made to the other account.
- Consistency
Data is in a consistent state when a transaction starts and when it ends.
For example, in an application that transfers funds from one account to another, the consistency property ensures that the total value of funds in both the accounts is the same at the start and end of each transaction.
- Isolation
The intermediate state of a transaction is invisible to other transactions. As a result, transactions that run concurrently appear to be serialized.
For example, in an application that transfers funds from one account to another, the isolation property ensures that another transaction sees the transferred funds in one account or the other, but not in both, nor in neither.
- Durability
After a transaction successfully completes, changes to data persist and are not undone, even in the event of a system failure.
For example, in an application that transfers funds from one account to another, the durability property ensures that the changes made to each account will not be reversed.
Change data - Transformations
Working on a holistic approach on information processing starting at the core activities can solve al lot of problems. Why just working on symptoms and not on root causes?
💡 Preparing data for BI, Analytics has become getting an unnecessary prerequisite. Build a big design up front: the enterprise data ware house (EDWH 3.0).
 
Data Technical - machines oriënted.
The technical machines oriënted approach is about machines and the connections between them (network).
The service of delivering Infrastructure (IAAS) is limited to this kind of objects. Not how they are inter related.
The problem to solve behind this are questions of:
- Any machine has limitations with performance.
❓ Consideration question: is it cheaper to place additional machines (* default action) or analysing performance issues by human experts.
- Confidentiality and Availability.
The data access has to be managed, backups and software upgrades (PAAS). All with planned outage times. Planning and coordination involved parties.
❓ Consideration question: is it cheaper to place additional machines (* default action) or manage additional complexity by human experts for machine support.
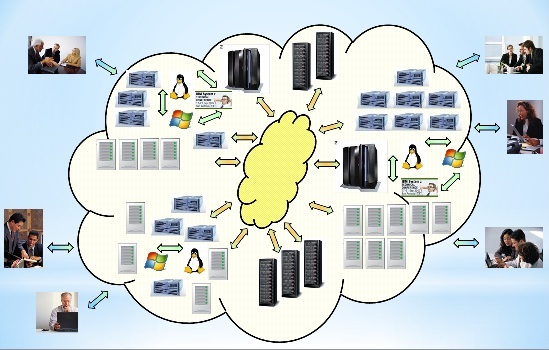
🤔 A bigger organisations has several departments. Expectations are that their work has interactions and there are some central parts.
Sales, Marketing, Production lines, bookkeeping, payments, accountancy.
🤔 Interactions with actions between all those departments are leading to complexity.
🤔 The number of machines and the differnces in stacks are growing fast. No matter where these logical machines are.
For every business service an own dedicated number of machines will increase complexity.
The information process flow has many interactions, inputs, tranformtions and outputs.
- ⚠ No relationsship machines - networking. The problem to solve that will popup at some point.
- ⚠ Issues by datatype conversions, integrity validation when using segragated sources (machines).
💡 Reinvention of a pattern. The physical logistic warehouse approach is well developed and working well. Why not copy that pattern to ICT? (EDWH 3.0)
What is delivered in a information process?
The mailing print processing is the oldest Front-end system using Back-end data. The moment of printing not being the same of the manufactured information.
Many more frontend deliveries have been created recent years. The domiant ones becoming webpages and apps on smartphones.
A change in attitude is needed bu still seeing it as a delivery needed the quality of infomration by the process.
Combined pages as single topic.
🕶 info types different types of information
✅ info types different types of data
👓 Value Stream of the data as product
👓 transform information data inventory
👓 data silo - BI analytics, reporting
🔰 Most logical
back reference.
© 2012,2020 J.A.Karman